Ron Dembo on using a unique science-based approach for generating forward looking scenarios
Ron Dembo from Riskthinking.AI talks to Climate Action about using a unique science-based approach for generating forward looking scenarios and how the product offerings differ from others in the field.
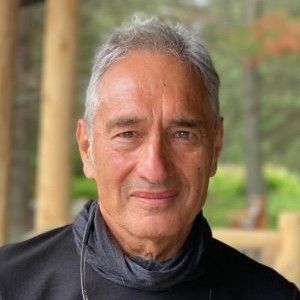
Ahead of the Sustainable Investment Forum Europe 2021, Ron Dembo from Riskthinking.AI talks to Climate Action about using a unique science-based approach for generating forward looking scenarios and how the product offerings differ from others in the field.
Riskthinking.AI data is based on a new climate standard that we have developed for climate financial risk measurement – the CLIMATE RISK CLASSIFICATION STANDARD (CRCS). One advantage is that it allows us to link Transition Risk with Physical risk in a completely coherent manner. We also generate multiple scenarios on combinations of multiple risk factors to evaluate the potential impacts on physical assets in the future. These aspects are unique to us.
Why is it important to generate scenarios that are multifactor and forward looking?
Most climate events are the product of multiple risk factors coming together in some particular way – think of fire risk; it is the product of particular combinations of the risk factors Heat, Drought and High Winds. To properly analyze impacts we need to be able to develop multiple scenarios on the possible combinations of these factors to capture the uncertainty in these impacts. We are unique at this level and use a patented algorithmic scenario generation method to derive scenarios. This also allows us to create consistent analyses for different geographies and different sectors.
Your ‘Climate Wisdom’ solution is an algorithmically devised climate risk data service- is this one of the most comprehensive climate risk data tools on the market and what makes it different?
As mentioned previously, we analyse risk using algorithmic based scenarios, based on multiple factors. We also link transition scenarios with their resulting physical risk impacts. The product is driven by climate science so we capture the fact that a climate driver, think of the El Nino affects different areas of the world in different ways but simultaneously. The correlation between the effects in one area and another are captured by us. All of this makes us unique.
Why is causality needed in data science and how can this benefit climate risk and ultimately financial risk? How do you model geographical causality into your data?
Because we are dealing with tremendous uncertainty in the future, uncertainty in science, uncertainty in policy etc correlations between risk factors and probabilities of their occurrence make no sense. The only thing we do have is the causality of climate science. Our product moves with the latest science.
Do you agree that climate stress test exercises should allow for a wide enough set of scenarios to limit the underestimation of losses?
Absolutely, in a very uncertain situation (radical uncertainty) we need to span the range of possible outcomes. Our algorithmic scenario generation method guarantees that we will find the best and worst scenarios in most circumstances.
Many countries, corporations and asset managers are pledging Net Zero Carbon. How can your software help them with this goal?
Net zero in 2050 is a long way away and many things could happen in between. It is a laudable goal but one that carries a lot of risk. Our risk thinking methodology and our data and stress testing analytics are great tools for dynamically managing the risk of meeting a net zero goal
Ron Dembo will be speaking at the Sustainable Investment Forum Europe in April, bringing together asset owners and managers, ratings agencies, banks, UN and Government policymakers, investors, development banks, think tanks, and NGOs committed to driving forward the sustainable finance agenda. Register your place for free here.